You signed in with another tab or window. Reload to refresh your session.You signed out in another tab or window. Reload to refresh your session.You switched accounts on another tab or window. Reload to refresh your session.Dismiss alert
Right now it appears that we're getting numerical overflows when casting to float16 in float32_to_int16 for some models (RWKV-LM, T0pp, Unified QA). These models were trained in bfloat16, not float16, which has a higher dynamic range and can represent larger numbers than float16 can.
bfloat16 has the nice property that its dynamic range is effectively the same as float32, it just has lower precision within that range. So we should essentially never convert large numbers into inf if we save everything in bfloat16.
So I'm proposing we save everything in bfloat16 across the board. Since this isn't supported natively by datasets or its Apache Arrow backend, we'd still use the reinterpret-as-int16 hack. The other option would be to choose which precision to use dynamically based on the precision of the model's weights, but I'd prefer not to deal with that complexity right now.
The text was updated successfully, but these errors were encountered:
Right now it appears that we're getting numerical overflows when casting to float16 in
float32_to_int16
for some models (RWKV-LM, T0pp, Unified QA). These models were trained in bfloat16, not float16, which has a higher dynamic range and can represent larger numbers than float16 can.bfloat16 has the nice property that its dynamic range is effectively the same as float32, it just has lower precision within that range. So we should essentially never convert large numbers into
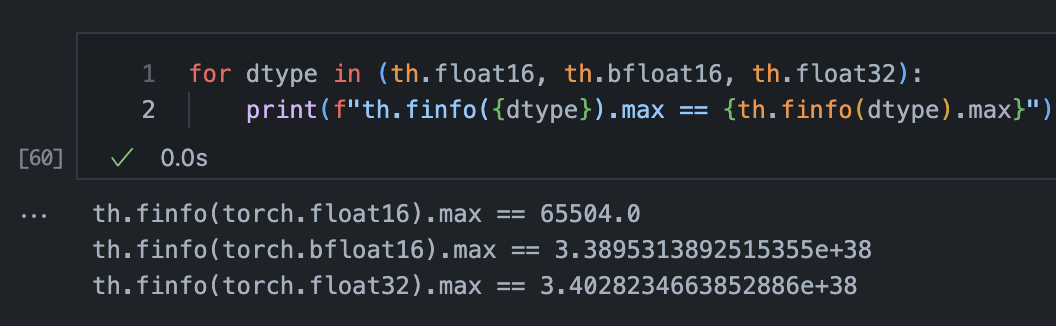
inf
if we save everything in bfloat16.So I'm proposing we save everything in bfloat16 across the board. Since this isn't supported natively by
datasets
or its Apache Arrow backend, we'd still use the reinterpret-as-int16 hack. The other option would be to choose which precision to use dynamically based on the precision of the model's weights, but I'd prefer not to deal with that complexity right now.The text was updated successfully, but these errors were encountered: